Automated Lexicon Generation
COVID-19 lexicon generation based on BERT model.
Natural Language Processing (NLP) models consisting of Bidirectional Encoder Representations from Transformers (BERT) have been used to extract emerging COVID-19 symptoms. However, limited research surrounding lexicon generation exists for acute and long-term COVID-19 symptoms (hereby referred to as “COVID-19 symptoms”) using NLP models from clinical notes to aid clinical decision-making. This becomes crucial as more deadly strains of COVID-19 emerge.
To assist in clinical decision-making by extracting sequences of lexical tokens for known COVID-19 symptoms from clinical notes, this study proposed an iterative approach to fine-tune a pre-trained BERT model for Named Entity Recognition (NER). The lexicon extraction performance of this fine-tuned BERT model was examined by using the extracted lexical tokens to annotate for the presence of mentions for six prevalent COVID-19 symptoms (dyspnea, fatigue, fever, cough, headaches, and nausea) on two manually-annotated reference corpora of notes.
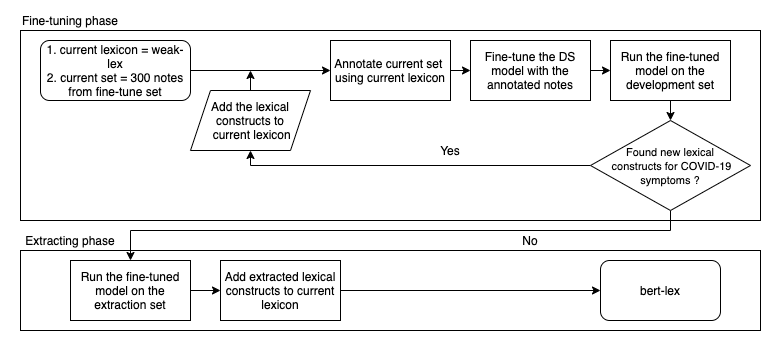
It was observed that the lexicon generated using BERT had similar f1-score compared to the lexicon generated only by clinical experts. Hence, the proposed methodology could substantially minimize valuable clinical content expert time spent on lexicon generation while maintaining quality through streamlining the process of generating lexicon of COVID-19 symptoms present in clinical notes.
Manuscript found here. Accepted as a poster at American Medical Informatics Association 2021.